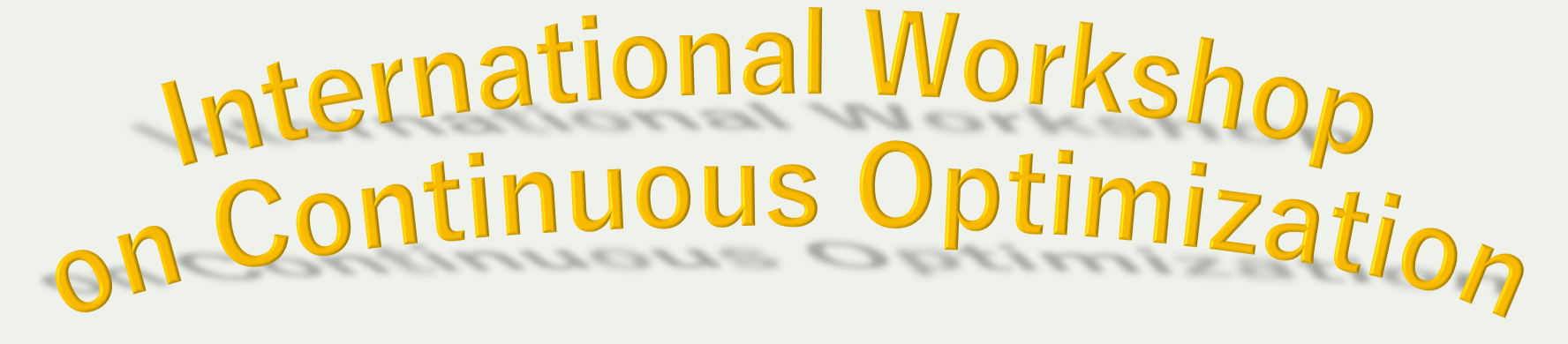
Schedule Overview
Note:: the schedule is JST (Japan Standard Time).
- December 3rd (Sat)
- 10:55-11:00, Opening Remarks
- 11:00-11:30, Vera Roshchina (The University of New South Wales)
- Some cones are particularly nice, but how nice exactly?
- 11:30-12:00, Sunyoung Kim (Ewha W. University)
- Exact Solutions of Quadratic Programs via Conic Relaxations
- 12:00-13:30,
- ++ Lunch Break ++
- 13:30-13:50, Godai Azuma (Tokyo Institute of Technology)
- Exactly Solving a class of QCQPs via Semidefinite Relaxation with Bipartite Sparsity Patterns
- 13:50-14:10, Mitsuhiro Nishijima (Tokyo Institute of Technology)
- Approximation Hierarchies for Copositive Cone over Symmetric Cone and Their Comparison
- 14:10-14:30, Takayuki Nagano (The University of Tokyo)
- Optimization of strongly convex function on hyperbolicity cone
- 14:30-14:50, Namchaisiri Charles (Tokyo Institue of Technology)
- An adaptation of Dual Spectral Projected Gradient Method
- 14:50-15:00,
- ++ Short Break ++
- 15:00-15:20, Atsushi Hori (Kyoto University)
- Two-stage distributionally robust noncooperative games: Existence of Nash equilibrium and its application to Cournot–Nash competition
- 15:20-15:40, Shotaro Yagishita (Chuo University)
- Exactness at Stationary Points of Sparse Constrained Problem
- 15:40-16:00, Issey Sukeda (The University of Tokyo)
- A study on modularity density maximization: Column generation acceleration and computational complexity analysis
- 16:00-16:20, Keita Kawakami (University of Tsukuba)
- Counterfactual Explanation Based on Mixed-integer Linear Optimization
- 16:20-16:30,
- ++ Short Break ++
- 16:30-17:00, James Saunderson (Monash University)
- Optimal self-concordant barriers for quantum relative entropies
- 17:00-17:30, João Gouveia (University of Coimbra)
- Self-dual polyhedral cones and their slack matrices
- December 4th (Sun)
- 10:00-10:30, Andreas Themelis (Kyushu University)
- Inertia and relative smoothness in nonconvex minimization: a case study on the forward-reflected-backward algorithm
- 10:30-11:00, Ching-pei Lee (Academia Sinica)
- Global convergence and acceleration of fixed point iterations of union upper semicontinuous operators: proximal algorithms, alternating and averaged nonconvex projections, and linear complementarity problems
- 11:00-11:10,
- ++ Short Break ++
- 11:10-11:30, Zihao Xiang (Tokyo Institute of Technology)
- An Exact Penalty Proximal DC Method for the Rank Constrained Quadratic Semidefinite Programming
- 11:30-11:50, Shota Takahashi (The Graduate University for Advanced Studies)
- Bregman Proximal DC Algorithms and Their Application to Blind Deconvolution with Nonsmooth Regularization
- 11:50-12:10, Kangming Chen (Kyoto University)
- A proximal gradient method with Bregman distance in multi-objective optimization
- 12:10-12:30, Naoki Marumo (University of Tokyo / NTT)
- Restarted accelerated gradient descent without knowledge of Lipschitz constants
- 12:30-14:00,
- ++ Lunch Break ++
- 14:00-14:30, Lei Yang (Sun Yat-sen University)
- Bregman Proximal Point Algorithm Revisited: A New Inexact Version and its Inertial Variant
- 14:30-15:00, Chengjing Wang (Southwest Jiaotong University)
- An efficient algorithm for the $\ell_{p}$ norm based metric nearness problem
- 15:00-15:10,
- ++ Short Break ++
- 15:10-15:40, Ting Kei Pong (Hong Kong Polytechnic University)
- Frank-Wolfe type methods for nonconvex inequality-constrained problems
- 15:40-16:10, Hiroyuki Sato (Kyoto University)
- General framework of conjugate gradient methods on Riemannian manifolds
- 16:10-16:20,
- ++ Short Break ++
- 16:20-16:40, Zhijian Lai (University of Tsukuba)
- Riemannian Interior Point Methods for Constrained Optimization on Manifolds
- 16:40-17:00, Sho Adachi (University of Tokyo )
- Riemannian Levenberg-Marquardt Method with Global and Local Convergence Properties
- 17:00-17:20, Hardik Tankaria (Kyoto University)
- Nys-LMN: Nyström Levenberg-Marquardt-type Newton
- 17:20-17:25, Closing Remarks
Title and Abstract
- December 3rd, 11:00-11:30, Vera Roshchina (The University of New South Wales)
- Some cones are particularly nice, but how nice exactly?
- The talk will be focussed on amenability and the relationship between this and other properties of convex cones. I will in particular talk about hyperbolicity cones and will mention some questions related to amenability and the facial structure of convex cones in general.
The work is based on collaboration with Bruno Lourenço and James Saunderson.
- December 3rd, 11:30-12:00, * Sunyoung Kim (Ewha W. University)
- Exact Solutions of Quadratic Programs via Conic Relaxations
- Quadratic optimization problems (QOPs) are known to NP-hard in general. Conic relaxation methods have been widely used to find an approximate solution of QOPs. The exact solution of QOPs by conic relaxations has been also studied to show that some classes of QOPs can be solved exactly. Semidefinite programming relaxations, second order cone
relaxations that are used for the exact solutions are numerically tractable, thus, the exact solutions of QOPs can be computed for the classes of QOPs, frequently with sign properties of the data matrices. The completely positive (CPP) relaxations also provide the exactness for a certain class of QOPs. As CPP relaxation is not numerically tractable, the study on CPP relaxations for the exact solution remains theoretical. We discuss methods to find the exact solutions of QOPs and present some recent developments.
- December 3rd, 13:30-13:50, * Godai Azuma (Tokyo Institute of Technology), Mituhiro Fukuda (Federal University of ABC), Sunyoung Kim (Ewha W. University), Makoto Yamashita (Tokyo Institute of Technology)
- Exactly Solving a class of QCQPs via Semidefinite Relaxation with Bipartite Sparsity Patterns
- We study sufficient conditions of quadratically constrained quadratic programs (QCQPs) to be exactly solved by their standard semidefinite programming (SDP) relaxations. Recently, conditions for QCQPs whose aggregated sparsity pattern graphs are forest has been proposed. In this talk, we focus on a wider class of QCQPs with bipartite sparsity structures, and we propose new exactness conditions under weaker assumptions than previous results. We also prove that using a certain conversion of QCQPs, our conditions cover another existing condition for QCQPs with the sign-definite property. Finally, several results given from our conditions including numerical experiments and some instances are discussed.
- December 3rd, 13:50-14:10, * Mitsuhiro Nishijima (Tokyo Institute of Technology), Kazuhide Nakata (Tokyo Institute of Technology)
- Approximation Hierarchies for Copositive Cone over Symmetric Cone and Their Comparison
- We first provide a sum-of-square-based inner-approximation hierarchy for the copositive (COP) cone over a general symmetric cone. We second provide a general framework of approximation hierarchies obtained by exploiting those for the usual COP cone. In particular, by exploiting polyhedral approximation hierarchies, we show that those for the COP matrix cone over the direct product of a nonnegative orthant and one second-order cone can be described by semidefinite constraints. In this case, we also compare them with the existing hierarchies. Combining the proposed approximation hierarchies with the existing ones, we can evaluate the optimal value of COP programming problems more accurately and efficiently.
- December 3rd, 14:10-14:30, * Takayuki Nagano (The University of Tokyo), Bruno F. Lourenço (The Institute of Statistical Mathmatics), Akiko Takeda (The University of Tokyo/RIKEN)
- Optimization of strongly convex function on hyperbolicity cone
- We propose a first-order method to optimize strongly convex functions with hyperbolicity cone constraints. The class of hyperbolicity cones has high expressive power and contains the semidefinite and symmetric cones as special cases. Until now, to solve hyperbolicity cone-constraint problems, interior point method (Guler, 1997) and gradient descent method using smoothing and acceleration techniques (Renegar, 2019) have been proposed, but these methods suffer from high computational costs per iteration. In this study, we propose a first-order approach based on the Frank-Wolfe method. Our proposed method uses the duality and geometric properties of the underlying hyperbolicity cone in order to achieve computationally cheap iterations.
- December 3rd, 14:30-14:50, * Namchaisiri Charles (Tokyo Institue of Technology), Liu Tianxiang (Tokyo Institute of Technology), Makoto Yamashita (Tokyo Institute of Technology)
- An adaptation of Dual Spectral Projected Gradient Method
- A Dual Spectral Projected Gradient Method (Dual SPG) proposed by Nakagaki et al. is a method for solving log-determinant semidefinite programming (Logdet-SDP), which appears frequently in many statistical models such as gaussian graphical models. This method has an interesting way of approaching the optimal solution based on projections on the intersection of box constraints and a set specified by a linear matrix inequality.
In this study, we modify the DSPG method to handle the Logdet-SDP with a hidden clustering structure, which has a complicated part that cannot be efficiently computed by the original DSPG due to the huge size of the matrix that represents this hidden clustering structure.
- December 3rd, 15:00-15:20, * Atsushi Hori (Kyoto University) and Nobuo Yamashita (Kyoto University)
- Two-stage distributionally robust noncooperative games: Existence of Nash equilibrium and its application to Cournot–Nash competition
- Two-stage distributionally robust stochastic noncooperative games with continuous decision variables are studied. In such games, each player solves a two-stage distributionally robust optimization problem depending on the decisions of the other players. Existing studies in this area have been limited with strict assumptions, such as linear decision rules, and supposed that each player solves a two-stage linear distributionally robust optimization with a specifically structured ambiguity set. This limitation motivated us to generalize and analyze the game in a nonlinear case. The contributions of this study are (ⅰ) demonstrating the conditions for the existence of two-stage Nash equilibria under convexity and compactness assumptions, and (ⅱ) consideration of a two-stage distributionally robust Cournot–Nash competition as an application, as well as an investigation into the conditions for the existence of market equilibria in an economic sense. We also report some results of numerical experiments to illustrate how distributional robustness affects the decision of each player in the Cournot–Nash competition.
- December 3rd, 15:20-15:40, * Shotaro Yagishita (Chuo University), Jun-ya Gotoh (Chuo University)
- Exactness at Stationary Points of Sparse Constrained Problem
- Nonconvex sparse optimization problems with the trimmed l1 norm (resp. truncated nuclear norm), which is a penalty function of cardinality (resp. rank) constraint, for sparse optimization have been actively studied. In this talk, we first introduce a unified framework that includes all the existing trimmed l1-penalized problems. We then show that under mild conditions, any d-stationary point of the generalized trimmed l1 (resp. truncated nuclear) penalized problem satisfies the corresponding constraint. This property is called “exactness” and our exactness result is superior to almost all existing results, especially from the viewpoint of practice. Finally, we show that we can obtain a d-stationary point of the problems by using algorithms based on the proximal mapping.
- December 3rd, 15:40-16:00, * Issey Sukeda (The University of Tokyo), Atsushi Miyauchi (The University of Tokyo), Akiko Takeda (The University of Tokyo)
- A study on modularity density maximization: Column generation acceleration and computational complexity analysis
- Community detection is a fundamental network-analysis primitive with a variety of applications in diverse domains. The modularity density is known to be an effective alternative to the well-known modularity. We first accelerate column generation for the modularity density maximization problem by employing a well-known strategy for dense subgraph discovery, called the greedy peeling. Moreover, we reformulate the auxiliary problem to a sequence of 0–1 linear programming problems, enabling us to compute its optimal value more efficiently and to get more diverse columns. Computational experiments using a variety of real-world networks demonstrate the effectiveness of our proposed algorithm. Finally, we show the NP-hardness of a slight variant of the modularity density maximization problem, where the output partition has two or more clusters, as well as showing the NP-hardness of the auxiliary problem.
- December 3rd, 16:00-16:20, * Keita Kawakami (University of Tsukuba), Yuichi Takano (University of Tsukuba)
- Counterfactual Explanation Based on Mixed-integer Linear Optimization
- Counterfactual explanation (CE) is a method to find "the easiest improvement action" to reverse the predicted result of a classifier to the desired result.
Among them, CE methods using mixed-integer linear optimization (MILO) formulations have an advantage of evaluating the ease of improvement actions while imposing complex constraints (e.g., the order of actions and combination with other features).
In this presentation, we consider some extensions of the distribution-aware counterfactual explanation (DACE) method, which was proposed to take account of feature correlations and risk of outliers.
We also discuss through computational experiments how to reduce computation time and incorporate nonlinear correlations of features.
- December 3rd, 16:30-17:00, Hamza Fawzi (University of Cambridge), * James Saunderson (Monash University)
- Optimal self-concordant barriers for quantum relative entropies
- Quantum relative entropies are jointly convex functions of two positive definite matrices that generalize the Kullback-Leibler divergence and arise naturally in quantum information theory. Self concordant barriers are well-behaved barrier functions on a convex cones that are a key ingredient in efficient interior point methods for solving conic optimisation problems with respect to that cone.
In this talk, I'll discuss joint work with Hamza Fawzi (Cambridge) that establishes the self-concordance of natural barrier functions for the epigraphs of various quantum relative entropies and divergences. Furthermore we show that these barriers have optimal barrier parameter (a measure of complexity of the barrier). The techniques we develop extend to give optimal self-concordant barriers for various closed convex cones related to the noncommutative perspectives of operator concave functions.
- December 3rd, 17:00-17:30, João Gouveia (University of Coimbra), Bruno F. Lourenço (ISM)
- Self-dual polyhedral cones and their slack matrices
- We analyze self-dual polyhedral cones and prove several properties about their slack matrices. In particular, we show that self-duality is equivalent to the existence of a positive semidefinite (PSD) slack. Beyond that, we show that if the underlying cone is irreducible, then the corresponding PSD slacks are not only doubly nonnegative matrices (DNN) but are extreme rays of the DNN matrices and, more surprisingly, we show that unless the cone is simplicial PSD slacks not only fail to be completely positive matrices but they also lie outside the cone of completely positive semidefinite matrices.
- December 4th, 10:00-10:30, * Andreas Themelis (Kyushu University), Ziyuan Wang (University of British Columbia), Hongjia Ou (Kyushu University), Xianfu Wang (University of British Columbia)
- Inertia and relative smoothness in nonconvex minimization: a case study on the forward-reflected-backward algorithm
- The use of momentum to accelerate convergence of first-order algorithms has been gaining renewed interest ever since its first appearance 60 years back. Initially inspired by the physics intuition that inertia is effective in preventing oscillatory behaviors, momentum-type techniques are typically designed in attempt to improve convergence speed. While this effect can only be achieved and justified for positive momentum coefficients, our study on the forward-reflected-backward splitting of Malitsky and Tam suggests the necessity of "negative" values to guarantee convergence under mere relative smoothness assumptions, for nonconvex problems. Our conclusions are in line with, and more pessimistic than, a similar conjecture of Dragomir et al. for the mirror descent algorithm.
- December 4th, 10:30-11:00, Jan Harold Alcantara (Academia Sinica), * Ching-pei Lee (Academia Sinica)
- Global convergence and acceleration of fixed point iterations of union upper semicontinuous operators: proximal algorithms, alternating and averaged nonconvex projections, and linear complementarity problems
- We propose a unified framework to analyze fixed point iterations of a set-valued operator that is the union of a finite number of upper semicontinuous maps, each with a nonempty closed domain and compact values. We discuss global convergence, local linear onvergence under a calmness condition, and component identification, and further propose acceleration strategies that drastically improve the convergence speed.
Our framework is applied to analyze a class of proximal algorithms for minimizing the sum of a piecewise smooth function and the difference between pointwise minimum of finitely many weakly convex functions and a piecewise smooth convex function. When realized on two-set feasibility problems, this algorithm class recovers alternating projections and averaged projections as special cases, and our framework thus equips these classical methods with global convergence and possibilities for acceleration on a broad class of nonconvex feasibility problems. By specializing the framework to a nonconvex feasibility problem reformulation of the linear complementarity problem, we show global convergence to a solution from any initial point, with a local linear rate, of the alternating projection as well as the averaged projection methods, which is difficult to obtain on nonconvex problems. We further discuss realizations of our framework on sparsity-constrained problems and exemplify sharper convergence rates of our acceleration schemes under various conditions, such as the KL condition or the second-order sufficient optimality condition. Experiments also show that our acceleration techniques provide significant efficiency improvement over existing methods.
- December 4th, 11:10-11:30, * Zihao Xiang (Tokyo Institute of Technology), Kazuhide Nakata (Tokyo Institute of Technology)
- An Exact Penalty Proximal DC Method for the Rank Constrained Quadratic Semidefinite Programming
- We consider the rank constrained quadratic semidefinite programming (RQSDP) with constraints consisting of a number of linear equality constraints, a rank constraint, and a positive semidefinite cone constraint. We first replace the rank constraint by adding a difference-of-convex (DC) penalty function in the objective and give a sufficient condition to ensure that this penalty problem is global exact with respect to the original problem. Next, for the penalty problem, we apply a proximal DC algorithm whose subproblem can be solved by the semismooth Newton-CG method (SNCG) with superlinear convergence. We also prove the convergence of the algorithm to a stationary point of the penalty problem. Finally, the efficiency of the algorithm is shown via numerical experiments.
- December 4th, 11:30-11:50, * Shota Takahashi (The Graduate University for Advanced Studies)
- Bregman Proximal DC Algorithms and Their Application to Blind Deconvolution with Nonsmooth Regularization
- Blind deconvolution is a technique to recover an original signal without knowing a convolving filter. It is naturally formulated as a minimization of a quartic objective function under some assumption. Because its differentiable part does not have a Lipschitz continuous gradient, existing first-order methods are not theoretically supported. In this presentation, we reformulate the objective function as a difference of convex (DC) functions and add nonsmooth regularization. Then, we apply the Bregman proximal DC algorithm (BPDCA) and the BPDCA with extrapolation (BPDCAe), whose convergences are theoretically guaranteed under the L-smooth adaptable (L-smad) property. BPDCAe outperformed other existing algorithms in image deburring applications.
- December 4th, 11:50-12:10, * Kangming Chen (Kyoto University)
- A proximal gradient method with Bregman distance in multi-objective optimization
- Recently, a multi-objective proximal gradient method was proposed, which is a suitable descent method for composite multi-objective optimization problems. However, the method solves subproblems using only Euclidean distances, and it requires the differentiable part of each objective to have a Lipschitz continuous gradient, which limits its application. We propose an extension of this method, by using Bregman distances, and requiring a less demanding assumption called relative smoothness. We also consider two stepsize strategies: the constant stepsize and the backtracking procedure. In both cases, we prove global convergence in the sense of Pareto stationarity, and analyze the convergence rate through some merit functions.
- December 4th, 12:10-12:30, * Naoki Marumo (University of Tokyo / NTT), Akiko Takeda (University of Tokyo / RIKEN)
- Restarted accelerated gradient descent without knowledge of Lipschitz constants
- We propose a restarted accelerated gradient method for minimizing nonconvex functions with Lipschitz continuous gradient and Hessian. The proposed method finds a solution where the gradient norm is less than ε in O(ε^(-7/4)) function and gradient evaluations. Unlike existing algorithms with the same complexity bound, our method requires no prior knowledge of the Lipschitz constants and the tolerance ε and thus is more practical. This practicality is achieved by estimating the Lipschitz constants with the help of a backtracking technique and two technical inequalities: a Jensen-type inequality for gradient and an error bound for the trapezoidal rule.
- December 4th, 14:00-14:30, * Lei Yang (Sun Yat-sen University)
- Bregman Proximal Point Algorithm Revisited: A New Inexact Version and its Inertial Variant
- In this talk, we focus on a general convex optimization problem, which covers various classic problems in different areas and particularly includes many optimal transport related problems arising in recent years. To solve this problem, we revisit the classic Bregman proximal point algorithm (BPPA) and introduce a new inexact stopping condition for solving the subproblems, which can circumvent the underlying feasibility difficulty often appearing in existing inexact conditions when the problem has a complex feasible set. Our inexact condition also covers several existing inexact conditions as special cases and hence makes our inexact BPPA (iBPPA) more flexible to fit different scenarios in practice. As an application to the standard optimal transport (OT) problem, our iBPPA with the entropic proximal term can bypass some numerical instability issues that usually plague the popular Sinkhorn's algorithm in the OT community. The iteration complexity of $O(1/k)$ and the convergence of the sequence are also established for our iBPPA under some mild conditions. Moreover, inspired by Nesterov's acceleration technique, we develop an inertial variant of our iBPPA, denoted by V-iBPPA, and establish the iteration complexity of $O(1/k^{\lambda})$, where $\lambda\geq1$ is a quadrangle scaling exponent of the kernel function. In particular, when the proximal parameter is a constant and the kernel function is strongly convex with Lipschitz continuous gradient (hence $\lambda=2$), our V-iBPPA achieves a faster rate of $O(1/k^2)$ just as existing accelerated inexact proximal point algorithms. Some preliminary numerical experiments for solving the standard OT problem are conducted to show the convergence behaviors of our iBPPA and V-iBPPA under different inexactness settings. The experiments also empirically verify the potential of our V-iBPPA for improving the convergence speed.
- December 4th, 14:30-15:00, * Chengjing Wang (Southwest Jiaotong University)
- An efficient algorithm for the $\ell_{p}$ norm based metric nearness problem
- Given a dissimilarity matrix, the metric nearness problem is to find the nearest matrix of distances that satisfy the triangle inequalities. This problem has wide applications, such as sensor networks, image processing, and so on. But it is of great challenge even to obtain a moderately accurate solution due to the $O(n^{3})$ metric constraints and the nonsmooth objective function which is usually a weighted $\ell_{p}$ norm based distance. In this paper, we propose a delayed constraint generation method with each subproblem solved by the semismooth Newton based proximal augmented Lagrangian method (PALM) for the metric nearness problem. Due to the high memory requirement for the storage of the matrix related to the metric constraints, we take advantage of the special structure of the matrix and do not need to store the corresponding constraint matrix. A pleasing aspect of our algorithm is that we can solve these problems involving up to $10^{8}$ variables and $10^{13}$ constraints. Numerical experiments demonstrate the efficiency of our algorithm.
In theory, firstly, under a mild condition, we establish a primal-dual error bound condition which is very essential for the analysis of local convergence rate of PALM. Secondly, we prove the equivalence between the dual nondegeneracy condition and nonsingularity of the generalized Jabocian for the inner subproblem of PALM. Thirdly, when $q(\cdot)=\|\cdot\|_{1}$ or $\|\cdot\|_{\infty}$, without the strict complementarity condition, we also prove the equivalence between the the dual nondegeneracy condition and the uniqueness of the primal solution.
- December 4th, 15:10-15:40, Liaoyuan Zeng (Zhejiang University of Technology), Yongle Zhang (Sichuan Normal University), Guoyin Li (University of New South Wales), * Ting Kei Pong (Hong Kong Polytechnic University)
- Frank-Wolfe type methods for nonconvex inequality-constrained problems
- The Frank-Wolfe method (also known as the conditional gradient method) implements efficient linear optimization oracles for minimizing smooth functions over compact convex sets. This method and its variants form a prominent class of projection-free first-order methods for a large variety of application problems such as matrix completion. In this talk, we extend this method to minimize smooth functions over a possibly nonconvex compact set, which is defined as the level set of a difference-of-convex function that satisfies mild regularity conditions. The key to our extension is the introduction of a new linear optimization oracle for the nonconvex compact set. We discuss convergence and present numerical experiments to illustrate the empirical performance of the proposed algorithm.
- December 4th, 15:40-16:10, * Hiroyuki Sato (Kyoto University)
- General framework of conjugate gradient methods on Riemannian manifolds
- Conjugate gradient methods are important first-order optimization algorithms both in Euclidean spaces and on Riemannian manifolds. However, while various types of conjugate gradient methods have been studied in Euclidean spaces, there are relatively fewer studies for those on Riemannian manifolds. In this talk, we discuss a novel general framework that unifies existing Riemannian conjugate gradient methods such as the ones that utilize a vector transport or inverse retraction. The proposed framework also develops other methods that have not been covered in previous studies. Furthermore, we clarify the global convergence properties of several specific types of algorithms in the proposed framework.
- December 4th, 16:20-16:40, * Zhijian Lai (University of Tsukuba), Akiko Yoshise (University of Tsukuba)
- Riemannian Interior Point Methods for Constrained Optimization on Manifolds
- We extend the classical primal-dual interior point methods from the Euclidean setting to
the Riemannian one. Our method, named the Riemannian interior point method (RIPM), is
for solving Riemannian constrained optimization problems. Under the standard assumptions, we establish locally superlinear and quadratic convergence of RIPM. Moreover, we prove the global convergence of RIPM with classical line search. These are generalizations of the classical frame of primal-dual interior point methods for nonlinear programming proposed by El-Bakry et al. in 1996.
- December 4th, 16:40-17:00, * Sho Adachi (University of Tokyo ), Takayuki Okuno (Seikei University), Akiko Takeda
(University of Tokyo)
- Riemannian Levenberg-Marquardt Method with Global and Local Convergence Properties
- We extend the Levenberg-Marquardt method on Euclidean spaces to Riemannian manifolds. Although a Riemannian Levenberg–Marquardt (RLM) method was proposed by Peeters in 1993, to the best of our knowledge, there has been no analysis of theoretical guarantees for global and local convergence properties. As with the Euclidean LM method, how to update a specific param- eter known as the “damping parameter” has significant effects on its perfor- mances. We propose a trust-region-like approach for determining the parameter. We evaluate the worst-case iteration complexity to reach an ε-stationary point, and also prove that it has desirable local convergence properties under the local error-bound condition. Finally, we demonstrate the efficiency of our proposed algorithm by numerical experiments.
- December 4th, 17:00-17:20, * Hardik Tankaria (Kyoto University), Nobuo Yamashita (Kyoto University), Dinesh Singh (Indian Institute of Technology Mandi), Makoto Yamada (Kyoto University / Okinawa Institute of Science and Technology).
- Nys-LMN: Nyström Levenberg-Marquardt-type Newton
- In this talk, we consider solving an optimization problem of minimizing a smooth and strongly convex function using the low-rank Hessian approximation. The recently proposed Nys-Newton method uses the Nyström approximation that computes a partial thin column Hessian of randomly selected parameters, then it uses the regularized Nyström to get a unique solution of search direction. However, the search direction may become unstable because of the low-rank structure when the regularized parameter is small. To get a stable search direction, we propose the Levenberg-Marquardt-type method for the Nys-Newton method. We provide the convergence analysis and present the numerical experiments on several benchmark datasets.